Uso de confiabilidade na rotulação de exemplos em problemas de classificação multirrótulo com aprendizado semissupervisionado
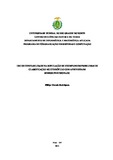
Visualizar/ Abrir
Data
2015-02-20Autor
Rodrigues, Fillipe
http://lattes.cnpq.br/4214726460957461
Metadados
Mostrar registro completoResumo
The techniques of Machine Learning are applied in classification tasks to acquire
knowledge through a set of data or information. Some learning methods proposed in
literature are methods based on semissupervised learning; this is represented by small
percentage of labeled data (supervised learning) combined with a quantity of label and
non-labeled examples (unsupervised learning) during the training phase, which reduces,
therefore, the need for a large quantity of labeled instances when only small dataset of
labeled instances is available for training. A commom problem in semi-supervised
learning is as random selection of instances, since most of paper use a random selection
technique which can cause a negative impact. Much of machine learning methods treat
single-label problems, in other words, problems where a given set of data are associated
with a single class; however, through the requirement existent to classify data in a lot of
domain, or more than one class, this classification as called multi-label classification.
This work presents an experimental analysis of the results obtained using
semissupervised learning in troubles of multi-label classification using reliability
parameter as an aid in the classification data. Thus, the use of techniques of
semissupervised learning and besides methods of multi-label classification, were essential
to show the results.